In this article, we will talk about the Azure service at OpenAI. We will start by presenting in broad strokes what Azure is and what types of generative AI models are present within the service, as well as how this service fits within Azure. Then, we will present various business use cases that have been reported by Microsoft, as well as use cases that we have identified at Devoteam, particularly in the field of data.
What is Azure OpenAI?
Have you heard about OpenAI and its recent developments in the media, including Chat GPT, GPT-3, and more recently GPT-4? OpenAI is a Californian company founded in 2015, originally as a non-profit organisation and later becoming a for-profit company in 2019. Among its founders is Elon Musk. The company’s objective is to develop and promote AI for the benefit of humanity. In 2019, Microsoft partnered with OpenAI to collaborate on the development of AI technologies, and to help OpenAI scale more quickly using the Azure platform.
The types of generative AI models in Azure OpenAI
So within Azure OpenAI, there are three types of models. The first type is generative text AI models, which include GPT-3 and the recently developed GPT-4. GPT-4 has the ability to be multimodal, meaning it can take both text and images as input. The second type is a descendant of the first, called “Codex”, which is also a natural language generation tool. This technology is used in “GitHub Copilot”. Finally, the third type is “Dall-E”, a family of generative AI models that can generate images from text.
How do generative AI models fit into Microsoft’s overall offering on Azure, specifically in the field of AI?
Microsoft’s platform, Azure, includes services that are accessible to both developers and business users. Users can create machine learning models from scratch using Azure Machine Learning or customise pre-trained models that are available in the Cognitive Services suite, of which OpenAI is a part. These services can then be reused in the Power Platform, which includes the Power Virtual Agent for building chatbots. Finally, there is a massive integration of these services within Microsoft 365 and Dynamics applications. For example, the release of Teams Premium allows for automatic meeting note generation, which is highly practical.
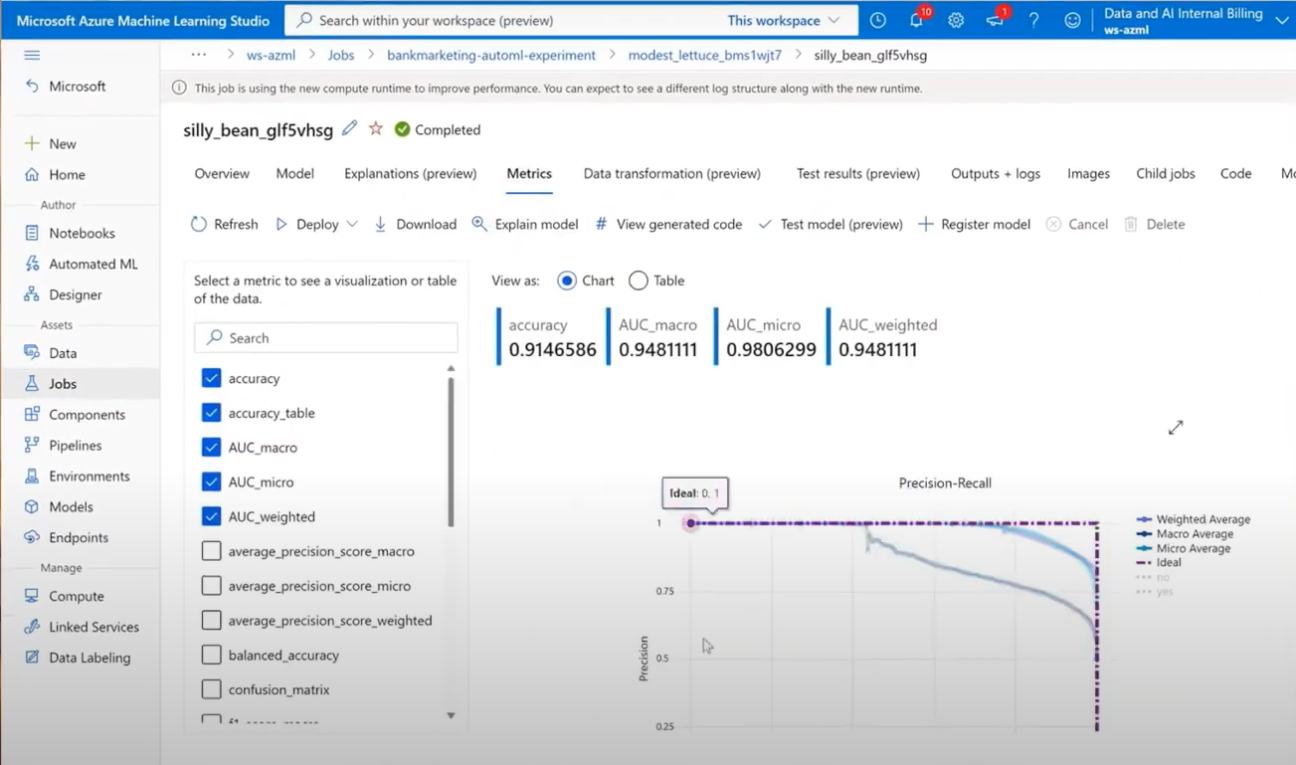
Azure Open AI: Business use cases
Before giving you specific examples of business use cases made possible by these technologies, I would like to highlight how these technologies should be approached. In my opinion, these technologies should be seen as a co-pilot, like in an airplane. It is a tool that will allow you to do your work better and more efficiently and to perform repetitive and tedious tasks more quickly. A study quantified the productivity gains generated by GitHub Copilot and showed that a developer who uses this technology develops 55% faster on average than a developer who does not use it. So, this technology will not replace you, (at least not yet), but it will allow you to gain productivity and focus on what is most essential in your work. For example, a user who is not familiar with a domain such as development or labour law will have difficulty understanding the correct commands in their tools and even more difficulty challenging the results that are returned. However, what is certain is that we will see a gap that will quickly develop between users who use these technologies and those who do not. Now that we have made this aside, let’s see together very concretely what are the business use cases made possible by these so-called generative technologies.
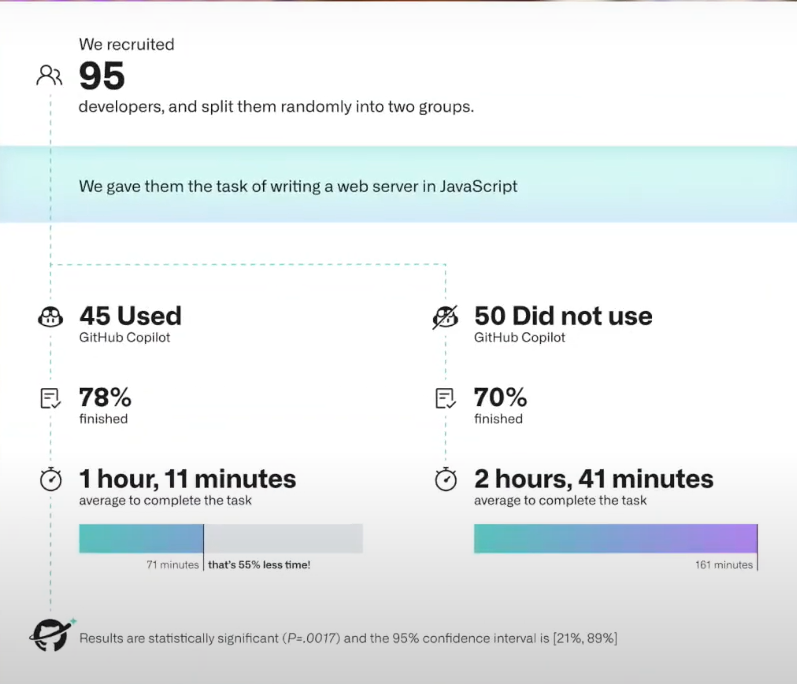
Let’s take a closer look at the specific use cases made possible by these generative AI technologies. You have probably already used each GPT in its web application, and you may have noticed that this service is capable of performing a multitude of tasks, including generating text, images, summaries, translations, and more. Microsoft has thus grouped the most common use cases reported by its clients and partners into four main categories.
Content generation
Firstly, there are use cases related to content generation. You can easily transform all the FAQs present on your web applications into chatbots to more efficiently answer your users’ questions. This can replace more traditional chatbots, which often struggle to deviate from their programmed responses and understand specific user requests. This will allow you to better respond to your users’ questions, particularly in call centres or support services that can offer live automatic translation, which is particularly useful for companies engaged in high-sourcing activities. The press has already reported on call centres that have used AI to eliminate accents from their operators, which is an intriguing prospect. Additionally, you can offer support service operators tools that will facilitate their search for answers for end users. You can also perform sentiment analysis based on recorded call transcriptions and even record a summary of the conversations. The possibilities are numerous.
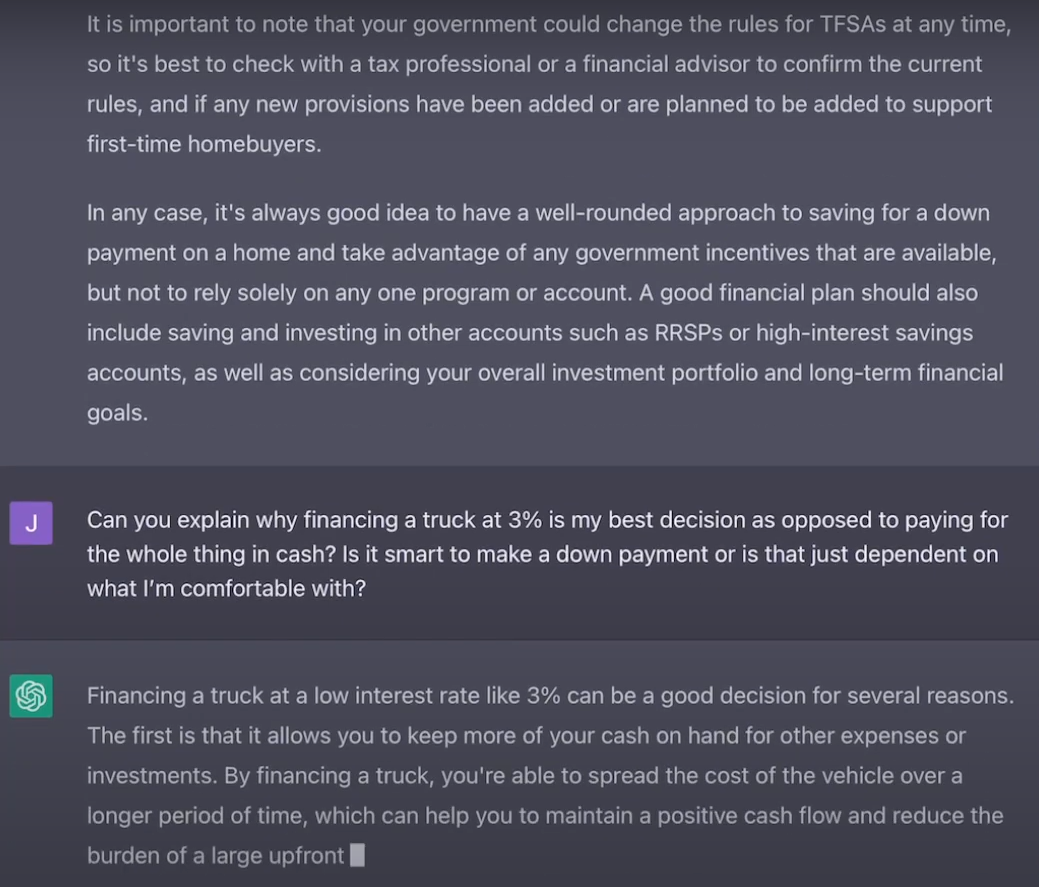
Summarization
Moving on to the second category, which is all about summarization. This is the second major group of use cases identified by Microsoft. With Chat GPT, you will be able to easily generate text summaries, which will be particularly useful in the finance industry, where it is common to summarise financial news reports, gather trends, and so on. It will also be possible, as I mentioned earlier, to summarise meetings, especially with Teams Premium. Additionally, there is the potential to automatically generate personalised training within your company on specific topics, which could be delivered via Fastrack mode. The possibilities are endless.
Code generation
The third major category is code generation. We talked about this earlier with tools like GitHub Copilot, which enhances developer productivity. However, this technology can also make programming languages and systems accessible to users who are more focused on business operations. This opens up the possibility of interactions between users and databases, where users can query a database in natural language, and the request is automatically translated into SQL query.
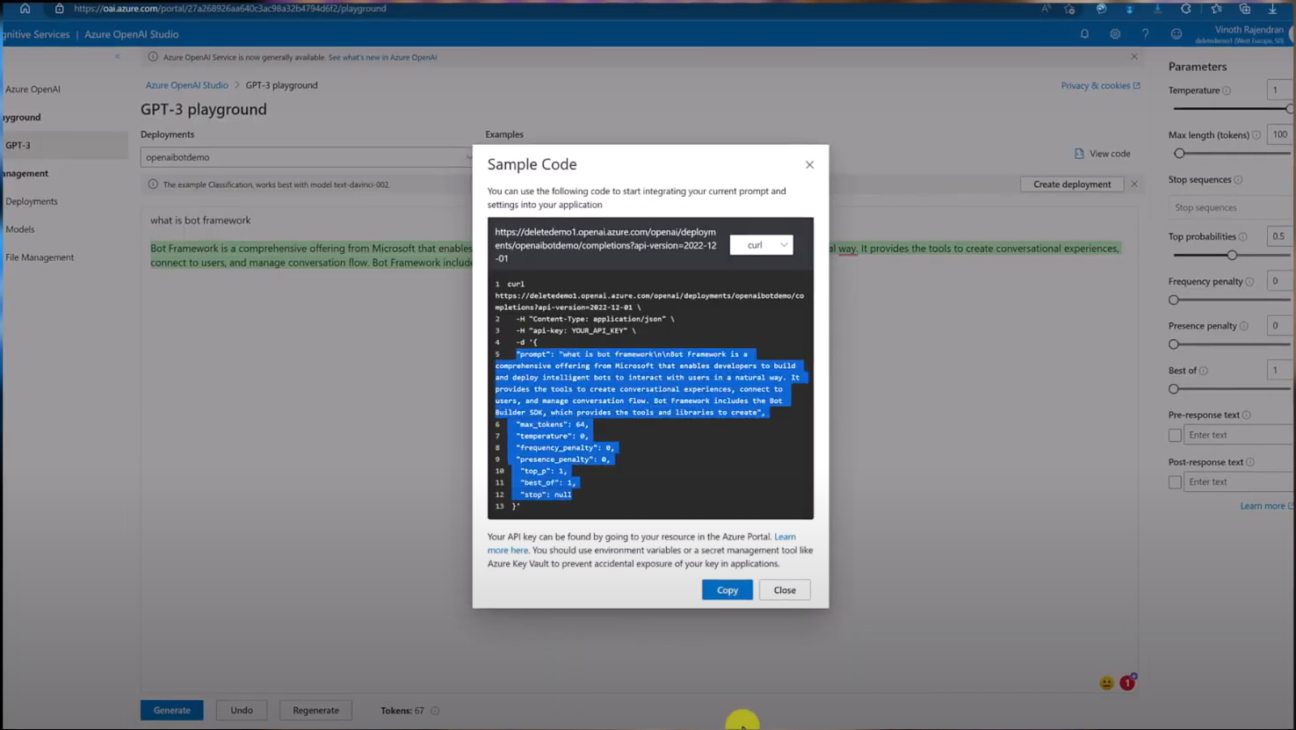
We also see the potential for automated documentation of code and even the generation of complete documentation from code. In the field of security, there are many use cases associated with this category of models, including the tool’s ability to detect vulnerabilities and flaws. For instance, the director of Coinbase recently used GPT-4 to scan the source code of Ethereum and discovered a critical vulnerability that had already been patched two years prior. This raises the question of whether the vulnerability was discovered by ChatGPT or if it simply highlighted old information that served as the basis for the model’s learning.
Semantic search
The fourth major use case category is semantic search. Just imagine the sheer volume of emails, Word documents, PowerPoint presentations, and PDFs that your organisation possesses. With this tool, you will be able to crawl through all this information to retrieve the right answers to all your questions. Essentially, you can think of this tool as a librarian. This category of use case models includes use cases for analysing contracts, terms, and conditions, among many other possibilities.
Other cases
So, more specifically, in the field of generic data or data science and BI, there are also numerous use cases. Here are some of the most interesting ones we noted and wanted to share with you regarding GPT-4. Firstly, there is the possibility of multimodal learning, which means that you can input images into the model and ask GPT-4 to analyse or comment directly on graphs, reports, or dashboards that were fed into the tool. Furthermore, by providing a basic database schema, GPT-4 can automatically generate a report or at least generate ideas for BI reports or visuals.
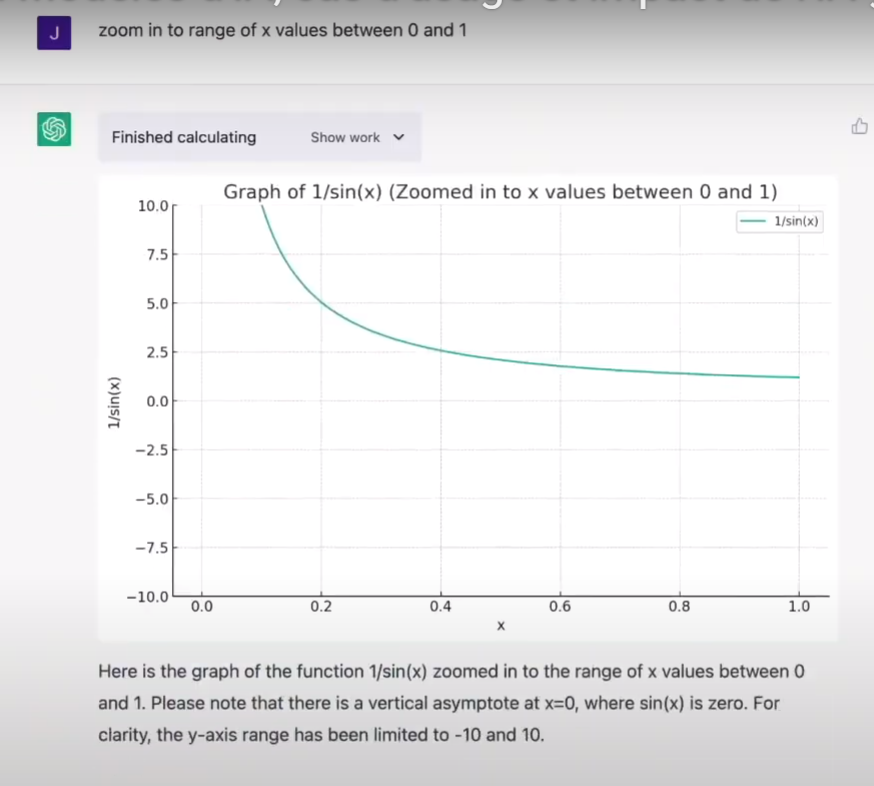
Another interesting use case is the possibility to facilitate code migrations. Specifically, if you need to convert an SQL query into Python code, we noticed that the tool performs very well in this task.
Another use case is to use the Dall-E family of generative image models to generate an image dataset that can be used to train other deep learning models. This is particularly useful when you do not have a large enough training set.
Additionally, you can use GPT-4 in brainstorming sessions to come up with ideas for interesting data use cases that can be realised within your organisation.
It’s clear that the possibilities are vast, and I’ve only scratched the surface by discussing these use cases. It’s up to you to explore the tool and discover how it can generate value within your organisation.